Brain and Computational Models in Learning: Explain
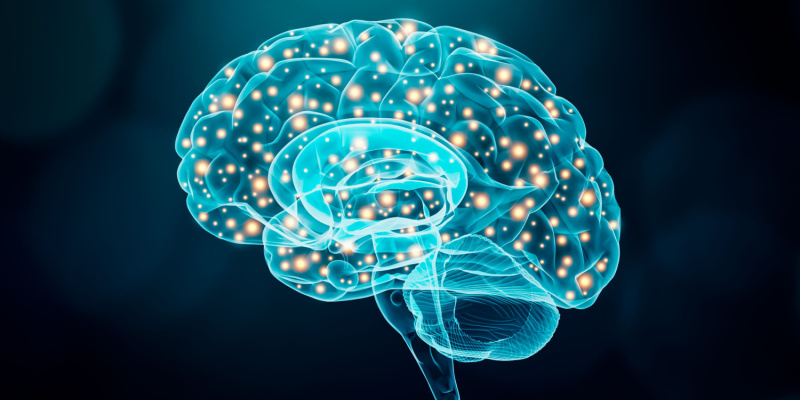
Brain and Computational Models in Learning: Explain
Under watchful eyes, machines with AI have grown fast to be a lot like our own brain, learning by doing and seeing things repeatedly. Big ideas from nature, like “Brain and Computational Models in Learning,” are now tucked under the hood of robots and gizmos with AI, potentially making them exceptionally proficient at their tasks. When we observe the similarities between brains and machines, it’s astonishing and enhances our ability to build intelligent robots.
Foundations of Learning Mechanisms
To get the idea of how learning works, we have to begin by understanding the basic rules used by both people’s brains and smart machines. People’s brains can get new knowledge because they have a bunch of tiny nerve cells mixed together. Like a wire web, these tiny cells hook up and share stuff they know. Smart machines have fake tiny nerve cell things too. They set these up in rows that let them get better at what they’re doing.
This is how stuff got known up to now (heretofore). In machines, lots of pretend cells make a kind of fake brain. Up to now, they act in ways that sort of mirror the brain in our heads. However, these pretend brains don’t work exactly like ours do. They kind of copy us, so that they can get new skills and get smarter over time. This surprise trick of growing know-how was unexpected and big for both brain studies and machine smarts.
Adaptation and Plasticity
Brains in living things and computer brains, like AI, can both change the way they work. This change happens when brain connections get either stronger or softer from what they go through, like learning something new. That’s called synapse change.
Computer brains are set up in their own way when they learn from stuff around them. They get better at figuring out shapes or intentions. Students’ Innovations in Generative AI at MIT Ignite ,Their learning shows how brains in heads and computer brains begin to work in much the same way.
It’s cool to see how both kinds of brains grow by adjusting. They both tune into learning twists in uncommon ways.
Finding Patterns in Chaos
In unguided teaching, both your brain and computer programs can spot connections or order in messy and unnamed info; they aren’t told what to look for but can find important stuff within a big jumble of data.
Isn’t it unusual that their ways of learning from confusion are quite alike?
Leveraging Past Knowledge
AI systems and human brains can both do something pretty nifty: when they learn a new fact or a trick in one area, it’s often useful for figuring stuff out in different but kind of similar spots – like knowing about dogs helps when learning about wolves later on. Many startling talents happen because of this helpful switcheroo; smart computer programs can actually copy how we think by using all the things they grasped before to get much better at new tasks. It’s just like getting the hang of clever moves by looking back at past mistakes or triumphs. In school, this same sneaky swap boosts how quickly someone can pick up on stuff they’ve never seen before.
Reinforcement Learning
Discover the intricate dynamics of “Brain and Computational Models in Learning.” Explore how reinforcement learning mirrors biological and artificial processes.Similarly, AI models receive feedback in the form of rewards or penalties, refining their strategies through successive iterations. This convergence underscores the shared fundamental principle of learning through experience.
Ethical Considerations in Converging Mechanisms
In the realms of AI and human brain studies, both aim for fair measures. Innovations seek harmony with good sense. A wise handle on concerns like hush-ups, slant, and owning up to deeds is needed, something heretofore not commonly faced in advancements’ history.
When new brain-like bots are made and set loose, things get tricky. This delicate mix sets deep questions front and Centre. As robot smarts and our own noodle’s ways entwine tighter, there’s a big need to think about doing it all upright and kind, so it does good for all of us, pretty much.
Challenges and Opportunities
Hard, it can be. Work, people must a lot. Beliefs powering techie thinking machines, with lessons from nature and Brain and Computational Models in Learning aren’t simple. Being mindful is unbelievably essential when such huge tasks are flipped and laid in scientists and inventors’ laps.
Gaps need to be filled. Head scratching pops up truly often. Not easily done, splitting thoughts into those for book-smarts and steps for widgets set the stage for seldom-seen surprises and tiresome crinkles in plans.
Conclusion
The convergence of learning mechanisms between the human brain and computational models marks a significant milestone in the development of AI. Exploring ‘Brain and Computational Models in Learning’ reveals parallels in foundational principles, adaptability, unsupervised learning, transfer learning, and reinforcement learning, highlighting shared approaches in biological and artificial intelligence. As we navigate the evolving landscape of AI, recognizing and harnessing this convergence opens the door to unprecedented advancements. The future of AI lies in our ability to draw inspiration from the intricate workings of the human mind, creating machines that not only simulate intelligence but also understand and learn in ways that echo the very essence of human cognition. The convergence of learning mechanisms is the bridge that connects these two realms, propelling us into a future where AI and human intelligence work hand in hand to push the boundaries of what is possible.